The MATLAB Curve Fitting Toolbox provides a user-friendly environment for fitting curves and surfaces to data, allowing for easy visualization and analysis of relationships within datasets.
Here’s a simple example of using the Curve Fitting Toolbox to fit a polynomial to data:
% Sample data
x = [1, 2, 3, 4, 5];
y = [2.3, 3.7, 5.1, 7.8, 11.2];
% Fit a polynomial of degree 2
fitResult = fit(x', y', 'poly2');
% Plot the data and the fitted curve
plot(fitResult, x, y);
xlabel('X-axis');
ylabel('Y-axis');
title('Curve Fitting with MATLAB');
What is Curve Fitting?
Definition
Curve fitting is a statistical technique used to create a curve that best represents the relationship between a set of data points. This can involve fitting simple lines (linear), complex curves (polynomial), or other forms of nonlinear functions to the data. The goal is to find a mathematical function that approximates the data while minimizing the difference between the actual data points and the values predicted by the curve.
Applications of Curve Fitting
The applications of curve fitting are vast and varied, making it an essential tool across multiple domains.
-
Science and Engineering: Curve fitting is used to model experimental data or phenomena, such as the relationship between temperature and pressure in thermodynamics.
-
Business and Economics: In market analysis, businesses often use curve fitting to forecast sales figures based on historical data trends.
-
Medicine and Biology: Researchers analyze biological data, such as dose-response curves, to model the effects of medication over time.
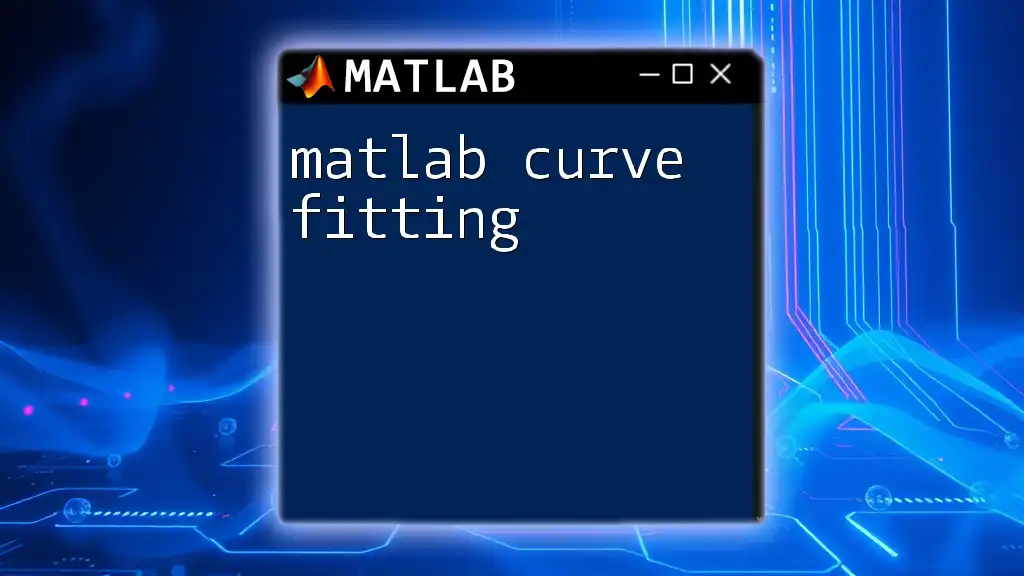
Getting Started with MATLAB Curve Fitting Toolbox
Installing the Toolbox
The first step in mastering the MATLAB Curve Fitting Toolbox is ensuring it is properly installed. To install the toolbox:
- Open MATLAB and navigate to the Add-Ons tab.
- Choose Get Add-Ons and search for "Curve Fitting Toolbox."
- Follow the prompts to install it.
To verify that the toolbox is available, use the following command in the MATLAB Command Window:
ver
This command will list all installed toolboxes. Look for Curve Fitting Toolbox in the output.
Accessing the Toolbox
Once installed, accessing the Curve Fitting Toolbox is straightforward. You can open the Curve Fitting App by typing the following command in the Command Window:
cftool
This command launches the Curve Fitting App, where you can visualize the data, select fitting models, and analyze the fitting results. The user interface presents options for importing data, selecting fit types, and customizing plots.
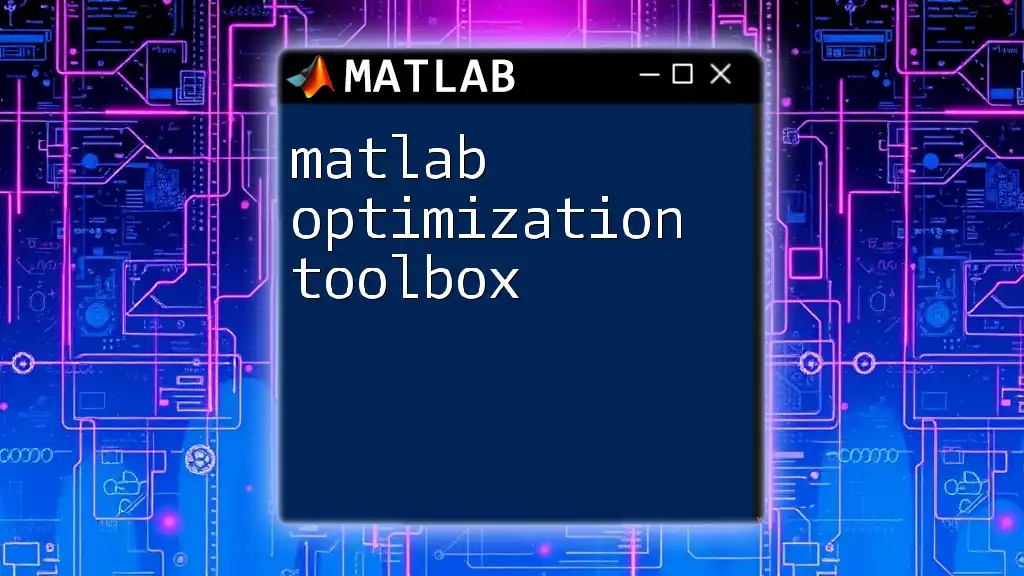
Basic Features of the Curve Fitting Toolbox
Creating Fittings
Importing Data
The first step in any fitting process is to import your data into MATLAB. This can be achieved by loading CSV files, Excel spreadsheets, or directly entering data arrays. An example of loading data from a CSV file is as follows:
data = readtable('mydata.csv'); % Assuming the first column is x and the second column is y
x = data.x;
y = data.y;
Selecting a Fit
Once the data is loaded, you need to choose a fitting type. MATLAB offers built-in models for linear and nonlinear fitting. For linear fitting, for example, you can select this option and simply click on the "Fit" button.
An example command for a linear fit in MATLAB would look like this:
ft = fit(x, y, 'poly1'); % Fitting a linear polynomial
Visualizing Fitting Results
Visualization is a powerful feature of the Curve Fitting Toolbox. After you have fit your model, you can plot the fitting results alongside the original data. Use the following command to plot:
plot(ft, x, y); % This will overlay the fit on the original data
You can customize the plot with titles, axis labels, and legends to enhance readability:
xlabel('X-axis Label');
ylabel('Y-axis Label');
title('Curve Fitting Result');
legend({'Data Points', 'Fitted Curve'});
Assessing Fit Quality
Goodness-of-Fit Measures
To determine how well your model fits the data, you need to assess the goodness-of-fit. Common measures include:
-
R-squared Value: Indicates how much of the variance in the data is explained by the model. Values close to 1 signify a good fit.
-
Residual Analysis: Plotting residuals (differences between observed and predicted values) helps diagnose potential fitting issues. An ideal residual plot should exhibit randomness.
You can calculate the R-squared value using:
RSquared = ft.rsquare; % Get R-squared from the fitting object
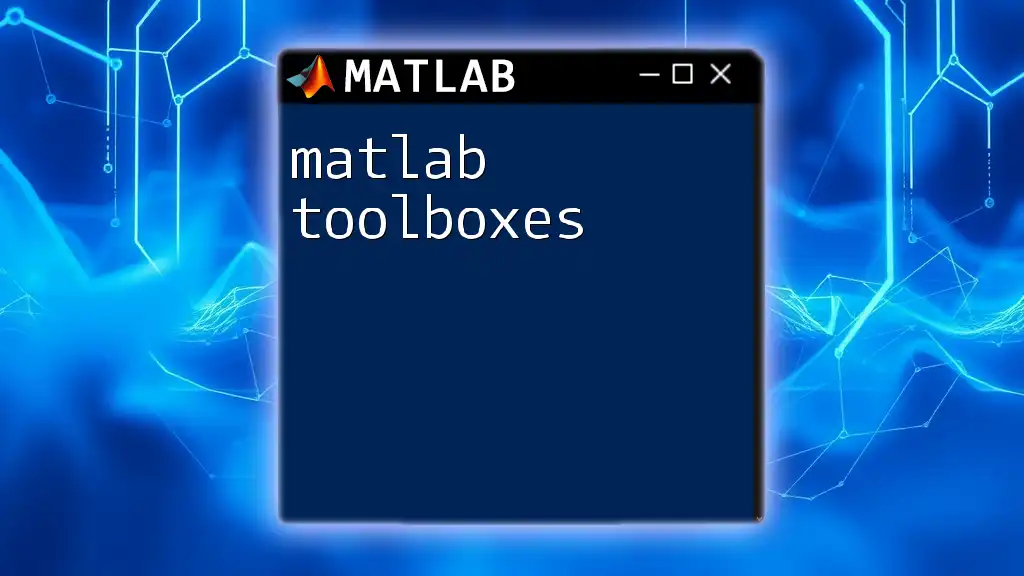
Advanced Curve Fitting Techniques
Custom Fitting Functions
For scenarios where built-in functions do not suffice, you can create custom fitting functions. Defining a custom function allows you to tailor the fitting process to your specific data characteristics. For instance, for a nonlinear model, you might define:
function y = customFitFunction(x, a, b, c)
y = a * exp(b * x) + c; % Example of an exponential fit
end
You can then use this function in the curve fitting process using:
ft = fit(x, y, @customFitFunction, 'StartPoint', [1, 0.1, 0]);
Fitting with Constraints
In some cases, you may want to impose bounds on your fitting parameters to yield more realistic results. MATLAB allows you to set these constraints easily. For example, to constrain parameter values during fitting:
% Constrain parameters, ensuring a >= 0 and b <= 1
opts = fitoptions('Method', 'NonLinearLeastSquares', 'Lower', [0, -Inf, -Inf], 'Upper', [Inf, 1, Inf]);
ft = fit(x, y, 'exp2', opts); % Fitting with constraints
Using the Fit Functions Programmatically
The Curve Fitting Toolbox enables programmatic access to fit functions, making it easy to integrate fitting into larger scripts and applications. For example, once you have constructed a fit, you can evaluate it over a range of values or generate new data points from the fit:
xfit = linspace(min(x), max(x), 100); % Generating values for fitting
yfit = feval(ft, xfit); % Evaluate the fitted function
By storing the fit coefficients, you can leverage them for further calculations or predictions.
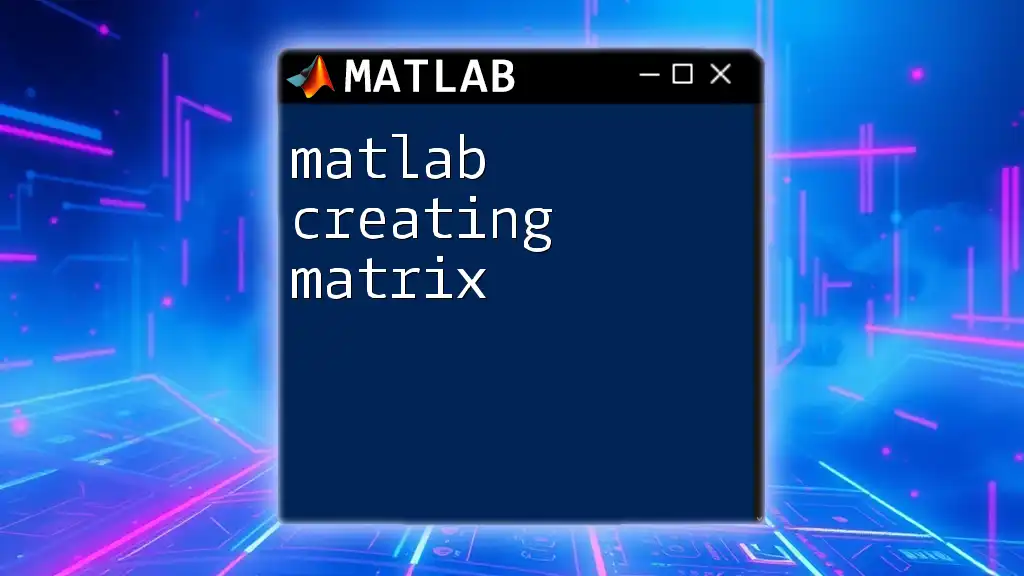
Curve Fitting in Practice
Case Study: Fitting a Polynomial to a Data Set
Consider an example where you have a dataset representing the height of plants over time. After importing and visualizing the data, you decide to fit a polynomial curve to model the growth.
Step-by-step, you can use:
x = [1, 2, 3, 4, 5]; % Time in weeks
y = [2, 3, 5, 10, 15]; % Height in cm
ft = fit(x', y', 'poly2'); % Fitting a second-degree polynomial
plot(ft, x, y); % Visualizing the fit
In this case, the polynomial fit provides an excellent approximation for the observed data, conveying the growth trend clearly.
Common Pitfalls
Curve fitting can sometimes lead to pitfalls such as overfitting or underfitting.
-
Overfitting occurs when a curve fits the noise in the data rather than the underlying trend. Inspect the residuals to ensure they do not exhibit patterns, which may indicate overfitting.
-
Underfitting happens when the chosen model is too simple to capture the complexities in the data. In such cases, experimenting with more complex models or transformations may yield better results.
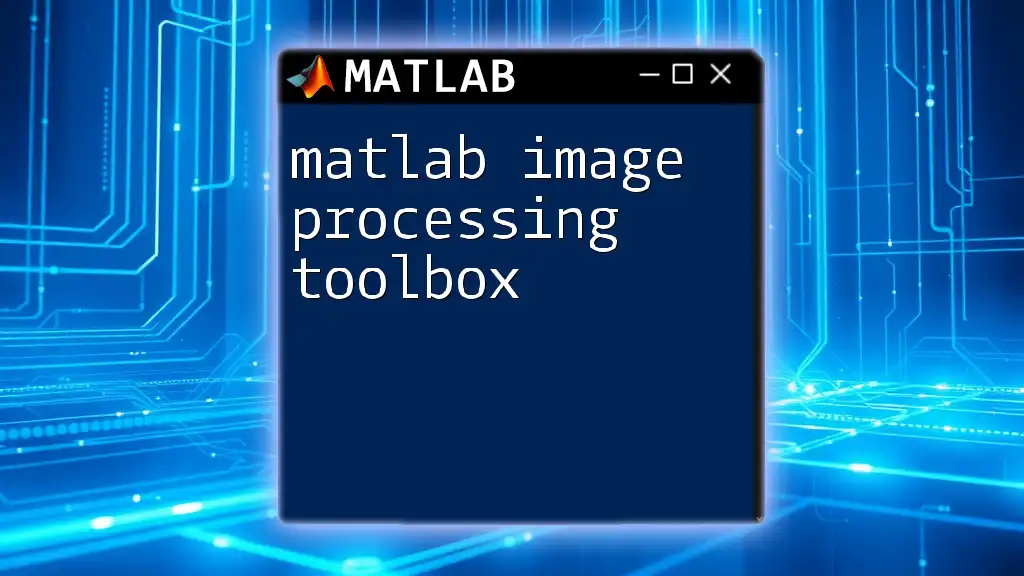
Conclusion
The MATLAB Curve Fitting Toolbox offers a powerful suite of tools for analyzing data and modeling relationships through curve fitting. From basic linear fits to advanced custom functions, this toolbox equips users with the capabilities to derive meaningful insights from their datasets. It is encouraged to practice and explore the various fitting techniques available.
To enhance your understanding and skills, consider diving into official MATLAB documentation or engaging with community resources, which can provide deeper insights and new learning opportunities.